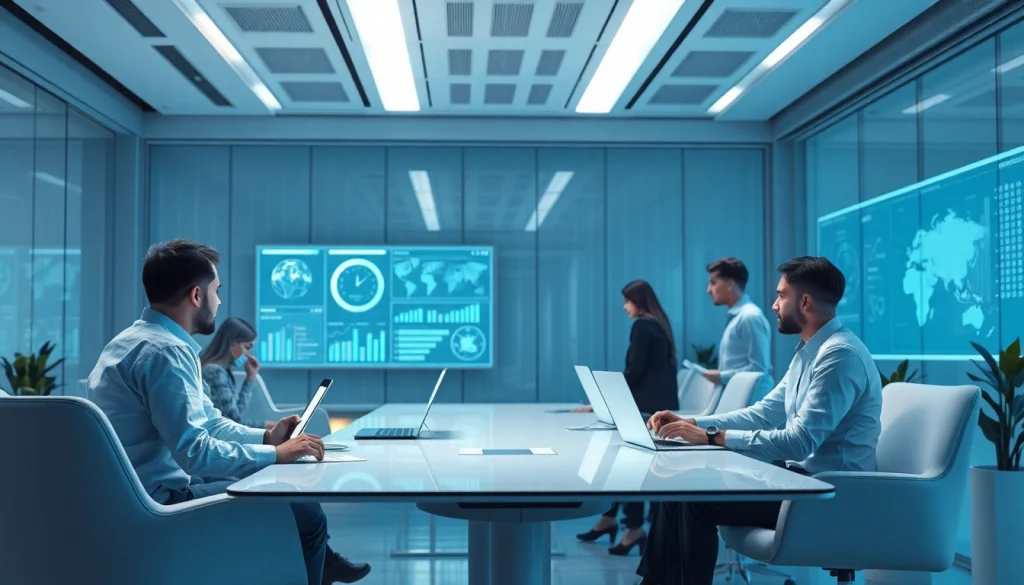
Introduction to Agentic AI
In an era where technology continues to evolve at an unprecedented rate, understanding the concepts that drive these advancements has never been more crucial. One of those concepts is Agentic AI, a burgeoning field of artificial intelligence focused on the autonomy of systems. It encapsulates AI technologies that not only process information but also learn from it, making decisions with minimal human intervention. This article aims to explore the intricacies of Agentic AI, comparing it to other forms of AI, discussing its advantages and disadvantages, and contemplating its future in various industry sectors.
Definition and Key Features
Agentic AI refers to sophisticated artificial intelligence systems designed to act independently, navigate complex environments, and solve intricate problems through autonomous decision-making processes. Unlike traditional AI, which often requires continuous human oversight, Agentic AI can adapt and evolve its strategies based on past experiences, real-time data, and shifts in external circumstances. Key features include:
- Autonomy: The capability to execute tasks without human guidance.
- Contextual Understanding: An ability to interpret varying environments and adjust actions accordingly.
- Iterative Learning: Continuous improvement of actions and strategies based on historical data and outcomes.
- Multi-step Problem Solving: Proficiency in addressing tasks that require a sequence of decisions and actions.
Historical Context and Evolution
The roots of Agentic AI can be traced back to earlier AI models that focused primarily on automation and data processing. Early AI systems were rigid, often performing predetermined tasks with little flexibility. The paradigm began shifting in the late 20th and early 21st centuries as researchers sought to create systems capable of dynamic learning and adaptation. This evolution was propelled by advancements in machine learning, neural networks, and computational power, allowing AI to progress from strictly deterministic operations to more autonomous frameworks.
Importance in Today’s Technology Landscape
In a technology landscape characterized by rapid change and increasing complexity, the importance of Agentic AI cannot be overstated. Businesses today require solutions that not only enhance operational efficiencies but also provide the agility to respond to rapidly shifting market demands. Agentic AI solutions can offer significant advantages across industries, from healthcare to finance to manufacturing. By automating decision-making processes, organizations can reduce costs, improve accuracy, and enhance customer satisfaction, thus positioning themselves competitively in their respective markets.
Differentiating Agentic AI from Other AI Types
Comparison with Generative AI
While both Agentic AI and Generative AI fall under the umbrella of artificial intelligence, their functions and objectives are fundamentally different. Generative AI is primarily focused on content creation—whether that be text, images, or music—by recognizing patterns and generating new outputs based on given inputs. On the other hand, Agentic AI emphasizes action and decision-making, aiming to achieve specific goals and objectives autonomously. In essence, Generative AI produces creative works, whereas Agentic AI targets operational tasks requiring strategic thinking and real-time responsiveness.
Understanding the Autonomy Aspect
One of the most defining characteristics of Agentic AI is its autonomy. Autonomous systems have the ability to operate independently, often in environments that are unpredictable or dynamically changing. This sets them apart from less advanced AI models, which may rely on extensive human supervision or predetermined pathways. Autonomous agents can assess their surroundings, make informed decisions based on real-time data, and adjust their strategies without waiting for human intervention. This capability is particularly valuable in fields like logistics, autonomous vehicles, and smart manufacturing, where rapid responses can lead to significant efficiencies.
Real-World Applications and Case Studies
The real-world applications of Agentic AI are vast and varied. In healthcare, for instance, Agentic AI can power diagnostic tools that autonomously interpret medical data and recommend treatment options, significantly reducing the time required for clinicians to make decisions. In finance, these systems can analyze vast amounts of transaction data to detect fraudulent activities or optimize investment portfolios. A case study exemplifying this is IBM’s Watson, which employs deep learning and predictive analytics to assist medical professionals in diagnosing diseases based on complex patient data.
Advantages of Implementing Agentic AI
Enhanced Efficiency and Decision-Making
Implementing Agentic AI can lead to significant enhancements in efficiency. By automating complex decision-making processes, organizations can minimize delays and reduce reliance on human input, which may introduce biases or errors. For example, in supply chain management, Agentic AI can forecast demand fluctuations and automatically adjust inventory levels, streamlining operations and reducing overhead costs.
Scalability and Adaptability in Different Industries
Agentic AI’s inherent adaptability makes it suitable for a breadth of industries. From retail and healthcare to transportation and finance, businesses can leverage these autonomous systems to scale operations seamlessly. As market conditions evolve, organizations deploying Agentic AI can quickly recalibrate their strategies without the need for manual intervention, driving growth and maintaining a competitive edge.
Reducing Human Error and Boosting Performance
Human error is an undeniable factor in many operational processes. Agentic AI systems reduce the margin for such errors by utilizing data-driven decision-making. In manufacturing, for example, these systems can monitor machine performance in real-time, predict failures before they occur, and optimize maintenance schedules, thus enhancing overall productivity and operational efficiency.
Challenges and Limitations of Agentic AI
Technological Hurdles in Deployment
Despite the numerous benefits, deploying Agentic AI is not without its challenges. Organizations often struggle with the integration of these systems into existing infrastructure. Technical hurdles such as data silos, legacy systems, and a lack of skilled personnel can impede the successful implementation of Agentic AI solutions. Additionally, the need for vast amounts of high-quality data can complicate deployment, as inaccuracies or inconsistencies in the training data can lead to suboptimal performance.
Ethical Considerations and Public Perception
As with any emerging technology, ethical considerations paint a complex picture for Agentic AI. Concerns about job displacement, data privacy, and the transparency of decision-making processes form the cornerstone of public perception challenges that organizations must navigate. As autonomous systems take on more responsibilities, maintaining public trust and ensuring compliance with ethical standards will be crucial for widespread acceptance.
Strategies to Overcome Limitations
To address the inherent challenges of deploying Agentic AI, organizations can adopt several strategies. Investing in workforce training can help bridge the skills gap, equipping employees with the knowledge necessary to work alongside autonomous systems. Furthermore, adopting an iterative approach to implementation—starting with pilot projects and gradually scaling—can help organizations troubleshoot potential issues without heavy upfront investment. Collaboration with AI experts and ethical committees can also provide guidance on best practices and ethical frameworks to follow.
Future Trends and Predictions for Agentic AI
Emerging Technologies and Innovations
The future of Agentic AI is poised for significant advances, particularly with the integration of supplementary technologies like Quantum Computing and advanced machine-learning algorithms. Innovations in these areas could enhance the processing capabilities of Agentic AI systems, enabling them to manage more complex scenarios and gather insights from larger datasets. Increased focus on explainable AI will also play a role; industries will demand systems that can articulate the reasoning behind their decisions to provide greater transparency.
Market Growth and Adoption Rates
According to market research, the demand for Agentic AI solutions is expected to surge in the coming years. Businesses are increasingly recognizing the competitive advantages of harnessing autonomous systems for decision-making, leading to greater adoption rates. This trend is expected to be amplified by initiatives in government and private sectors focused on digital transformation. Companies leveraging Agentic AI can expect to see improved operational efficiencies, increased revenue, and enhanced customer satisfaction levels.
Preparing for an AI-Driven Future
As Agentic AI continues to evolve, organizations must proactively prepare for an increasingly AI-driven future. Beyond technological adjustments, this preparation should encompass organizational culture shifts to embrace innovation and continuous learning. Developing frameworks that emphasize collaborative interactions between humans and AI, as well as fostering an adaptive mindset among employees, will be crucial for navigating the complexities of this new reality.