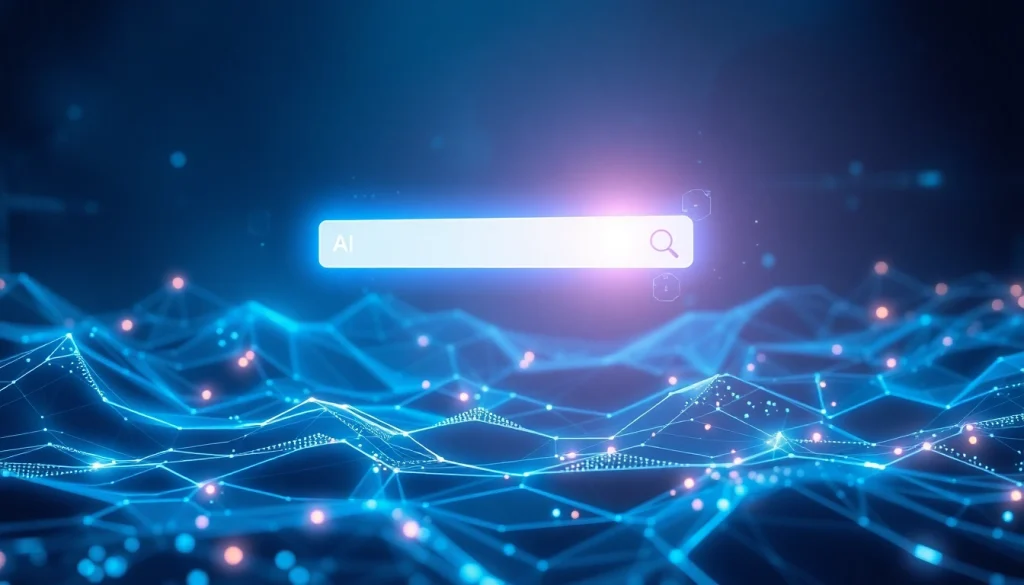
Introduction to AI Search
What is AI Search?
AI search refers to the integration of artificial intelligence technologies with search engines to enhance the search experience for users. Unlike traditional search methods that rely predominantly on keyword matching to return results, AI search leverages machine learning and natural language processing to understand the context and intent behind user queries. This allows for more relevant and nuanced results, often incorporating various data sources, real-time updates, and personalized content.
The Importance of AI in Modern Searching
With the exponential growth of information available online, the role of AI in search engines has become increasingly significant. AI-driven search engines can sift through vast amounts of data more efficiently than traditional engines. They provide a more tailored experience by learning user preferences over time, thereby adapting to their searching habits. The advent of AI search not only enhances user experience but also drives engagement and satisfaction.
How AI Search Differs from Traditional Search Engines
Traditional search engines typically rely on algorithms that use keyword density and backlinking as keys to ranking websites. AI search, on the other hand, uses advanced algorithms that consider multiple factors such as user intent, natural language processing, and contextual understanding. For example, while a traditional search might return pages based solely on the presence of keywords, AI search can provide results that better match the user’s query based on context, synonyms, and other semantic factors.
Key Features of Efficient AI Search Engines
Understanding Natural Language Processing in AI
Natural Language Processing (NLP) is a critical component of AI search technology. It enables search engines to interpret and generate human language in a way that is meaningful and contextually relevant. The NLP capabilities allow users to input queries in natural language, and the search engine to provide responses that align with user expectations, even if the wording differs from conventional keyword searches. This leads to a more conversational search experience.
Real-Time Data Access and Retrieval
Real-time data access is another paramount feature of AI search engines. Unlike traditional search engines, which may rely on a fixed set of indexed data that can become outdated, AI-driven search engines are designed to pull information from various sources, including news articles, social media, and more, updating their databases continuously. This ensures users receive the most current information available, particularly relevant in fields such as finance or technology, where change occurs swiftly.
User Personalization and Customization
User personalization is a hallmark of AI search, enhancing the overall experience by tailoring results based on individual user behavior and preferences. AI algorithms assess factors such as previous searches, interaction patterns, and other metrics to curate results that are likely to be of interest. This customization not only improves the relevance of search results but also fosters ongoing engagement as users are more likely to find valuable content consistently.
Comparative Analysis of AI Search Engines
Top AI Search Engines to Consider
As we look towards 2025 and beyond, a plethora of AI search engines is emerging, each offering unique features and advantages:
- Google’s Gemini: This AI search engine integrates various AI features and is renowned for its capability to process and understand visual content, making it a frontrunner.
- Microsoft’s Copilot: Positioned to enhance office productivity, Copilot integrates AI search capabilities to streamline document management and information query.
- ChatGPT Search: Leveraging OpenAI’s technology, this search engine delivers conversational responses and relevant links, transforming user interaction with search results.
- Perplexity: Known for its straightforward design and focus on providing factual clarity, Perplexity stands out with its user-friendly interface.
Pros and Cons of Using AI Search Engines
Just like any technology, AI search engines have their advantages and potential drawbacks:
Pros:
- Enhanced Relevance: AI search engines deliver more precise and contextually relevant results, improving user satisfaction.
- Efficiency: They can retrieve and process data faster than traditional methods.
- Personalization: Offers tailored experiences based on user data.
Cons:
- Privacy Concerns: The data collection necessary for personalization can raise privacy issues.
- Citation Challenges: The accuracy of information can be compromised if AI systems do not cite sources correctly.
- Dependency on Algorithms: Users may become overly reliant on AI systems, potentially diminishing critical thinking skills.
Case Studies of Successful AI Search Implementations
Real-world examples illustrate the effectiveness of AI search engines:
- Enterprise AI Search: Numerous businesses have adopted AI-driven search solutions to improve internal knowledge management, resulting in enhanced employee productivity and quicker project turnaround times.
- E-commerce Platforms: Many online retailers utilize AI search to refine product recommendations based on user behavior, leading to increased sales and improved customer satisfaction.
Challenges in AI Search Technology
Citation and Information Credibility Issues
One of the most pressing challenges facing AI search engines is the issue of citation credibility. As AI algorithms pull information from various sources, they often do so without clearly attributing the information. This can lead to misinformation and erode trust in AI-generated content.
User Privacy and Data Security Concerns
AI search engines often rely on extensive user data to tailor search results effectively. This reliance raises significant privacy issues, as users may be unaware of the extent of data collected or how it is used. Companies must navigate the balance between effective personalization and user privacy.
Algorithm Bias and Its Impact on Search Results
Algorithm bias remains a critical issue in AI search technology. If the algorithms are trained on biased data, they may inadvertently produce skewed results, which could reinforce stereotypes or misinformation. Addressing this bias is crucial for developing trustworthy AI search engines.
The Future of AI Search
Emerging Trends in AI Search Technology
The landscape of AI search is continually evolving. Emerging trends include increased integration of multimodal inputs (text, voice, and images) that allow search engines to understand and process information in more complex ways. Additionally, advancements in AI ethics will shape how algorithms are designed to reduce bias and enhance transparency.
Preparing for a Shift in User Search Behavior
As AI search technologies mature, user behavior will naturally shift. Users are likely to become accustomed to conversational search interfaces, which may influence how businesses and content creators optimize their material for search visibility. Preparing for this shift will be crucial for maintaining relevance in digital marketing strategies.
Recommendations for Businesses Adopting AI Search
For businesses looking to implement AI search solutions, several best practices are recommended:
- Focus on User Experience: Ensure that AI search tools improve the user experience rather than complicate it.
- Prioritize Data Ethics: Establish clear data usage policies that respect user privacy and comply with regulations.
- Invest in Algorithmic Transparency: Develop strategies to ensure algorithms are free from bias, providing thorough documentation of how searches are processed.